Nyamata, Bugesera, Iburasirazuba, RWANDA
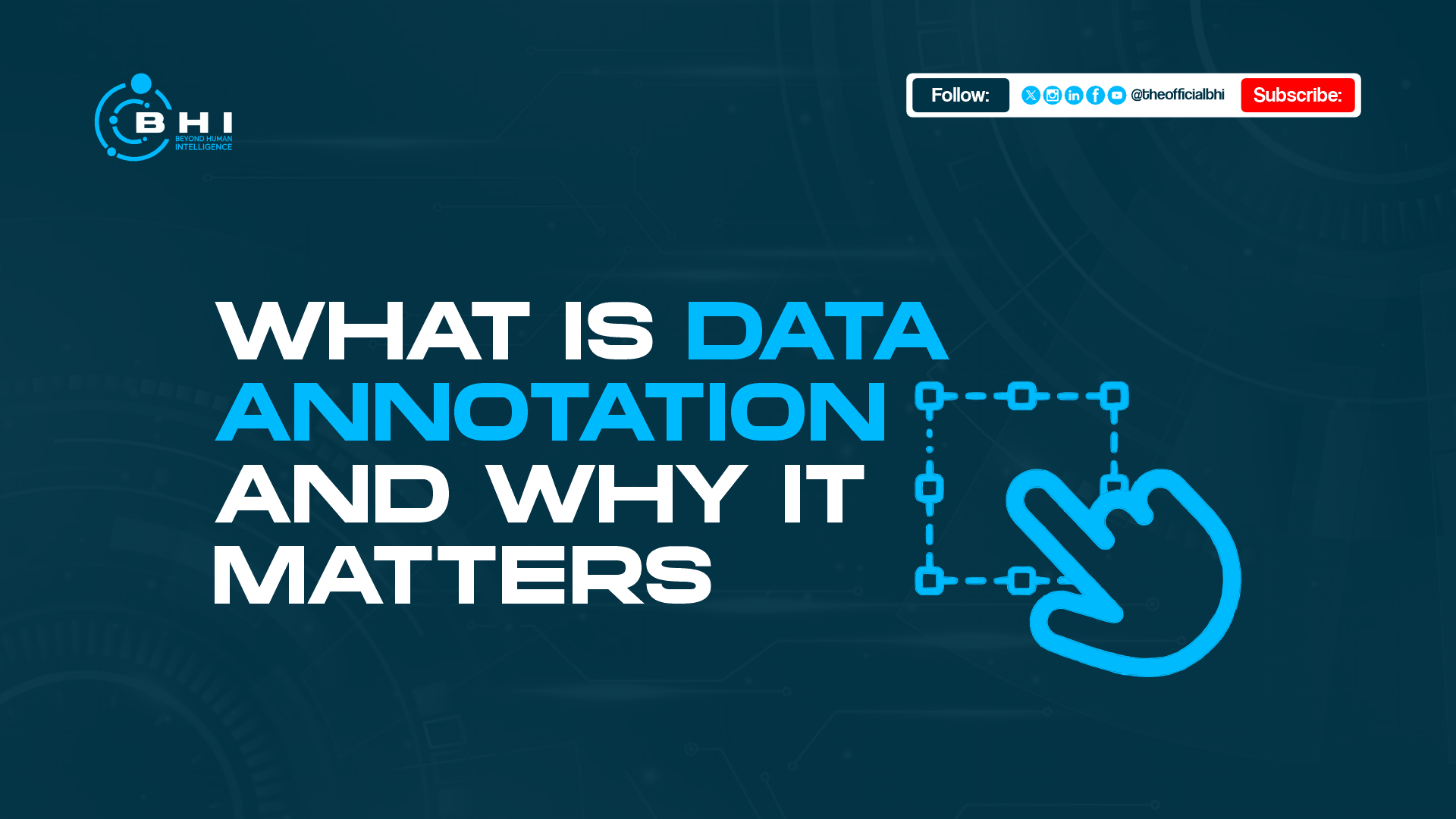
Artificial Intelligence (AI) sounds futuristic, but it’s already part of your daily life. From unlocking your phone with your face to chatting with customer service bots, you’re interacting with AI systems more than you think. But here’s something most people don’t realise:
AI doesn’t learn on its own. People teach it, and they do it through data annotation.
In this post, we’re breaking down:
- What Data Annotation?
- Why it’s the Secret Sauce Behind AI
- Common Types of Annotation
- Where You’ve Probably Seen the Results Without Knowing
Let’s dive in.
What Is Data Annotation?
At its core, data annotation means adding labels, tags, or instructions to raw data, like text, images, audio, or video, so that machines can understand and learn from it. Think of it like teaching a child. If you show a child 100 pictures of animals and point to each one, saying “dog,” “cat,” or “goat,” the child starts recognising them on their own. AI works the same way, but instead of human intuition, it needs thousands (or millions) of clearly labelled examples. In short, annotation is how we train AI.
Why Is It Important?
Data annotation is the foundation of almost every AI application you can think of. Without labelled data, AI would be just a powerful engine with no fuel.
Imagine this:
You want a model to detect potholes on Nigerian roads using drone footage. But the model doesn’t know what a pothole looks like. You now need to label thousands of images—this is a pothole, this is not. Only then can the model start learning patterns.
The same applies in:
- Healthcare (e.g., labelling X-rays or ultrasound images)
- Finance (e.g., tagging fraud patterns in transactions)
- Agriculture (e.g., identifying plant diseases from leaf images)
- Security (e.g., recognising faces or license plates)
No annotation = no learning.
Types of Data Annotation (With Everyday Examples)
Let’s explore some of the most common types, with examples you may recognise:
-
Image Annotation
Labelling objects in pictures. Example: Drawing a box around a car, a person, or a traffic light in a photo. Used in: Self-driving cars, facial recognition, and agricultural apps.
-
Text Annotation
Highlighting or categorising parts of text. Example: Tagging “angry” or “positive” in product reviews. Used in: Chatbots, translation tools, sentiment analysis.
-
Audio Annotation
Marking words, sounds, or emotions in audio files. Example: Tagging where a speaker says “hello” or when someone laughs. Used in: Virtual assistants, transcription software, and call centre analysis.
-
Video Annotation
Tracking movement or labelling actions across frames. Example: Following a footballer on the field during a match replay. Used in: Sports analytics, surveillance, and gesture recognition.
-
3D Point Cloud Annotation
Used for 3D data from LIDAR sensors (common in robotics). Example: Labelling buildings and trees in a drone’s 3d scan of a town. Used in: Mapping, autonomous navigation.
Each of these annotation tasks needs people—human eyes, ears, and judgment. AI doesn’t know what a smile means or how sarcasm works unless someone teaches it first.
Where You’ve Seen It (Without Knowing)
You’ve likely interacted with data annotation without realising:
Those annoying CAPTCHA tests (“click all the images with traffic lights”)? That’s you helping to annotate data. Netflix recommending your next binge? That’s labelled viewing behaviour. Instagram filtering spam comments? That’s based on tagged text data. AI tools get “smart” because people helped them learn by labelling patterns over time.
Why You Should Care
If you’re in tech, education, content creation, or just navigating a digital world, understanding data annotation gives you insight into:
- How AI decisions are made
- Where bias and error can creep in (because poorly labelled data = poor AI)
- New career and Freelance opportunities (annotation is growing fast, especially in remote workspaces)
- Plus, as more startups and companies in Africa and beyond build AI tools, there’s a growing need for quality Data, and that begins with annotation.
In Summary, data annotation might not be flashy. You won’t see it trending on Twitter or making headlines. But without it, AI simply doesn’t work.